๐ Predictive Analytics in Marketing Explained Simply
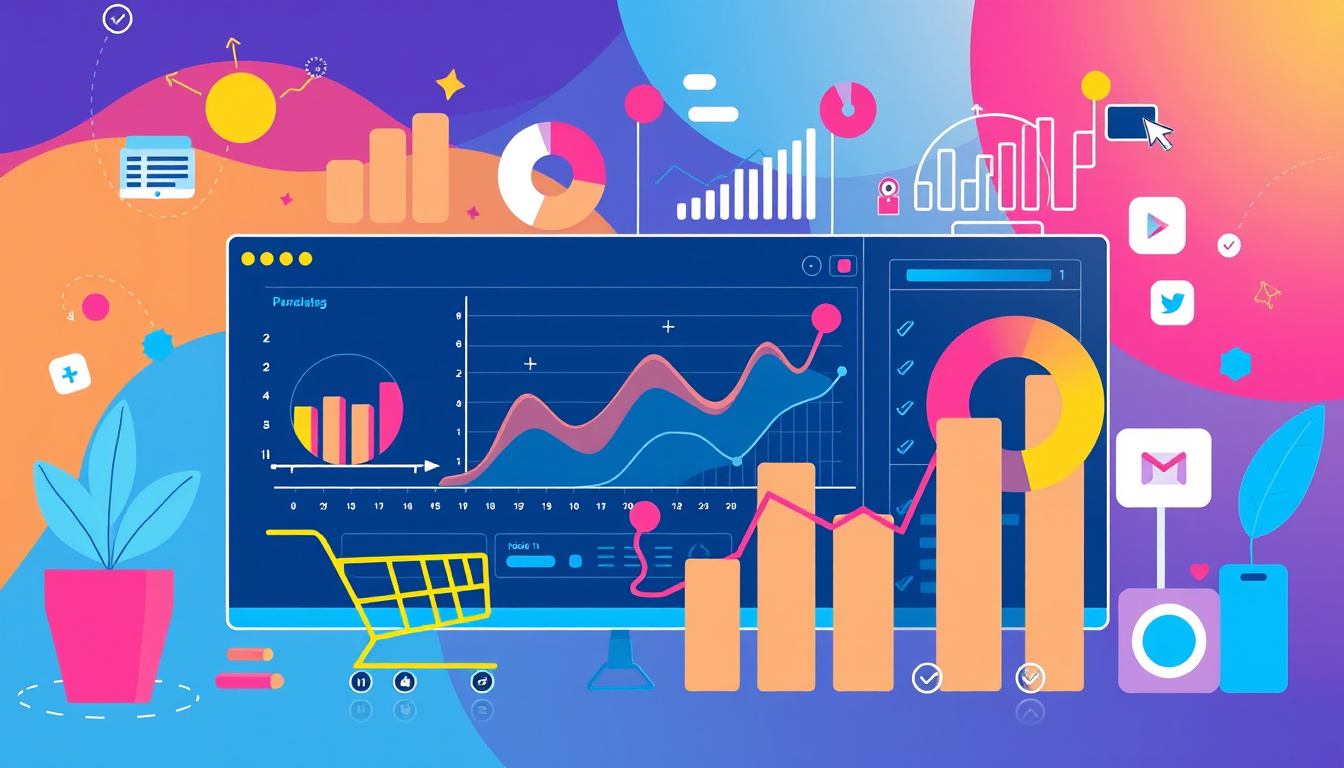
In todayโs competitive landscape, understanding consumer behavior is paramount. Enter ๐ Predictive Analytics in Marketing Explained Simply. This powerful approach utilizes data, statistical algorithms, and machine learning techniques to identify the likelihood of future outcomes based on historical data. Businesses can harness predictive analytics to improve decision-making, tailor marketing strategies, and enhance customer engagement. In this article, weโll explore what predictive analytics is, its importance for businesses, how it works, essential tools, real-world applications, and the challenges it presents, helping you grasp the significance of this innovative analytical method in marketing.
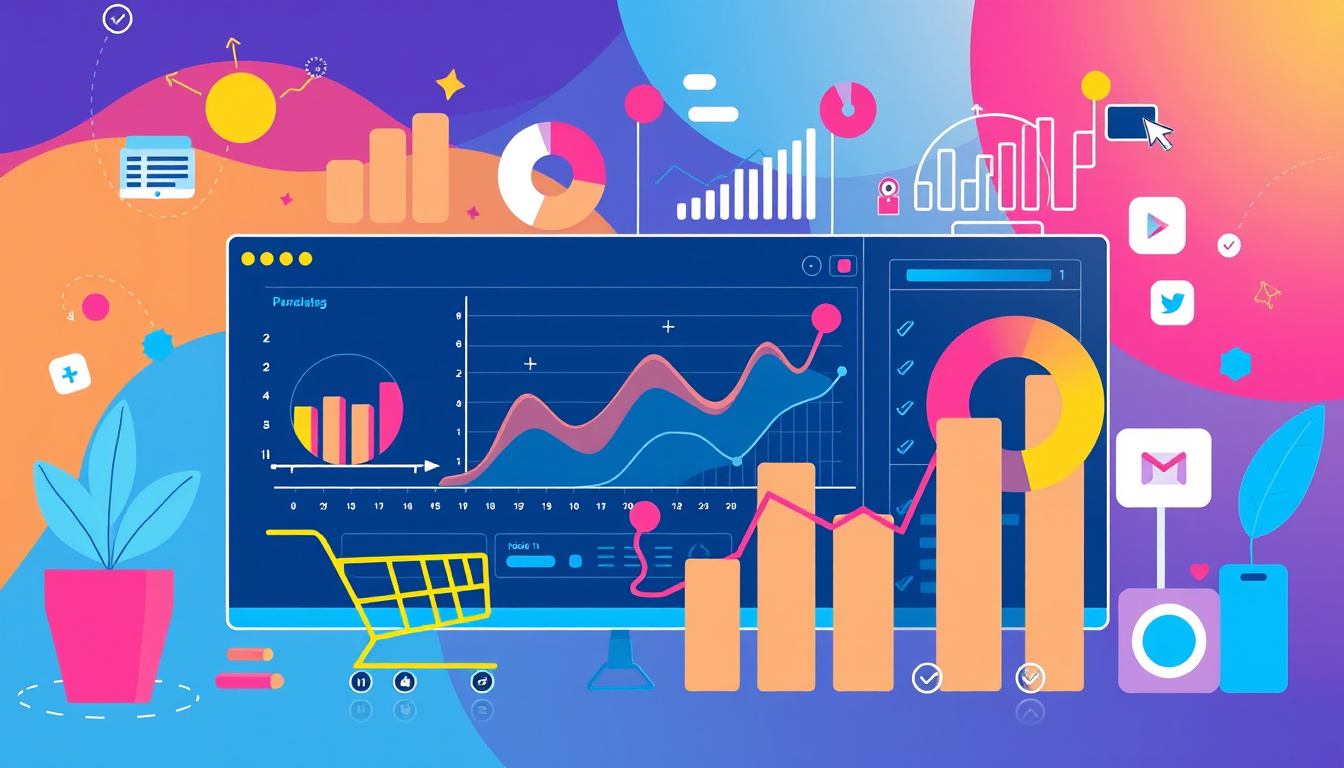
Key Takeaways
- Predictive analytics uses historical data to forecast future marketing outcomes.
- Understanding predictive analytics is crucial for businesses to make data-driven decisions.
- Key tools in predictive analytics include machine learning algorithms and data visualization software.
- Real-world examples demonstrate the effectiveness of predictive analytics in enhancing marketing strategies.
- Challenges such as data quality and privacy concerns must be addressed in predictive analytics implementations.
What is Predictive Analytics in Marketing?
Predictive analytics in marketing is a powerful tool that enables businesses to anticipate future consumer behaviors based on historical data and trends. This involves using statistical algorithms and machine learning techniques to analyze patterns within large datasets. By leveraging predictive analytics in marketing, companies can identify potential customers, tailor their marketing strategies, and improve ROI. For instance, a retailer may use predictive analytics to forecast which products are likely to be popular based on previous purchasing trends, seasonal behaviors, and even social media sentiment. This strategic foresight allows marketers to optimize their campaigns, engage customers in a timely manner, and improve overall customer satisfaction. With the ever-increasing volume of data generated in todayโs digital landscape, understanding predictive analytics in marketing explained simply helps demystify how brands can effectively harness data for smarter decision-making.
The Importance of Predictive Analytics for Businesses
In today's fast-paced business environment, leveraging data is crucial for staying competitive, and this is where ๐ predictive analytics in marketing explained simply comes into play. Predictive analytics involves using statistical algorithms and machine learning techniques to analyze historical data and make informed predictions about future trends and consumer behaviors. For businesses, this means not only understanding what has happened in the past but also anticipating what might happen next. By harnessing the power of predictive analytics, companies can tailor their marketing strategies effectively, optimize their resource allocation, and enhance customer engagement. Ultimately, integrating predictive analytics into marketing enables businesses to create personalized experiences for their customers, boost sales, and drive growth, ensuring they stay ahead in the ever-evolving marketplace.
'In the world of marketing, the best predictor of future behavior is past behavior.' โ Philip Kotler
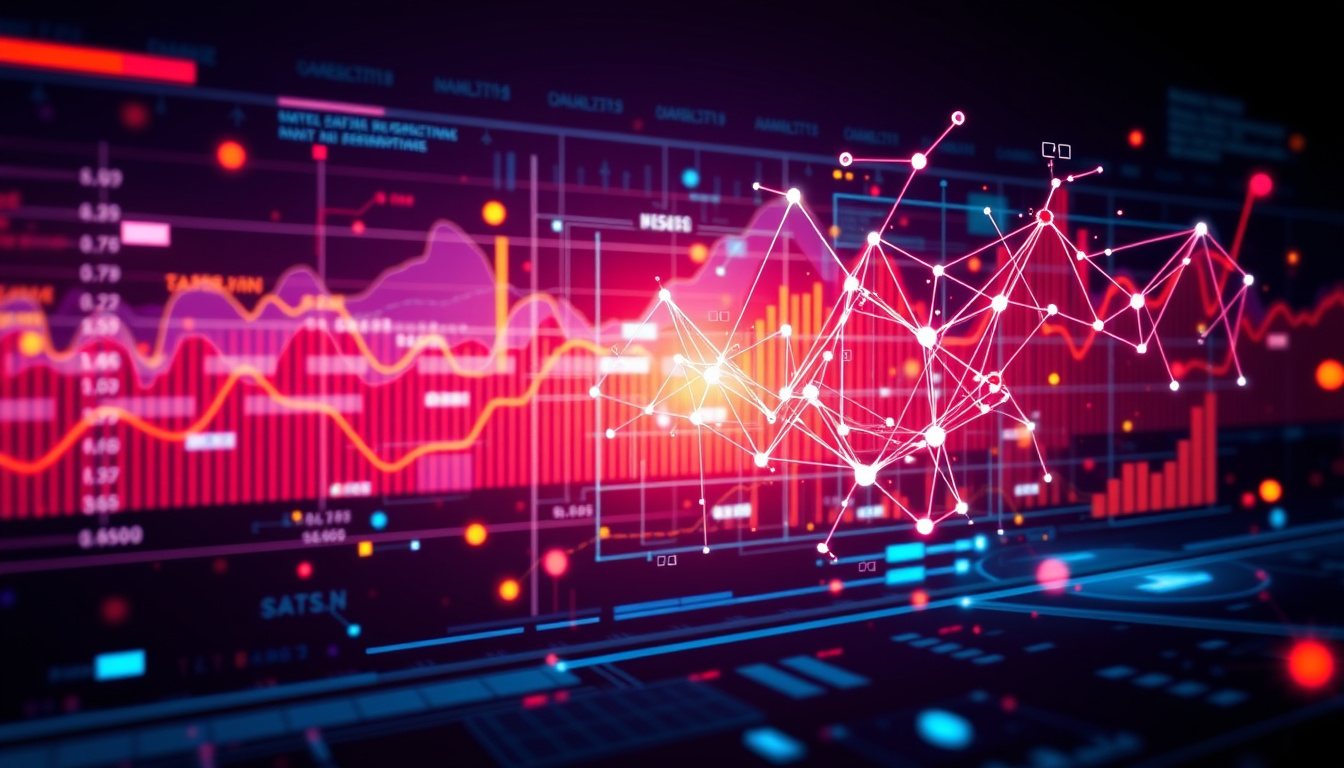
How Predictive Analytics Works: A Simple Breakdown
Predictive analytics in marketing explained simply revolves around utilizing historical data, statistical algorithms, and machine learning techniques to identify the likelihood of future outcomes based on past behaviors. At its core, predictive analytics functions through a systematic process: first, it collects relevant data from various sources, such as customer interactions, purchase history, and demographic information. Next, this data undergoes cleansing and preparation to enhance its quality for analysis. Once the data is ready, statistical models and machine learning algorithms are applied to identify patterns and trends that can forecast customer behavior, sales trends, and campaign effectiveness. The insights derived from predictive analytics empower marketers to make informed decisions, personalize customer experiences, and optimize marketing strategies, ultimately leading to greater ROI and customer satisfaction. By embracing this powerful tool, businesses can not only anticipate customer needs but also stay ahead of market competitors.
Key Tools and Techniques Used in Predictive Analytics
When it comes to leveraging data for strategic decision-making, ๐ predictive analytics in marketing explained simply can feel overwhelming, yet itโs more accessible than it seems. Key tools such as regression analysis, machine learning algorithms, and data mining techniques play crucial roles in this field. For instance, regression analysis helps marketers understand the relationships between variables, providing insights into what actions may yield the best results. Additionally, machine learning algorithms sift through vast datasets to identify patterns, allowing businesses to predict consumer behavior with greater accuracy. Data mining, on the other hand, involves extracting useful information from large datasets, which can uncover hidden trends that influence marketing strategies. By utilizing these tools effectively, marketers can not only forecast future trends but also personalize customer experiences, making predictive analytics an invaluable asset in today's data-driven marketing landscape.
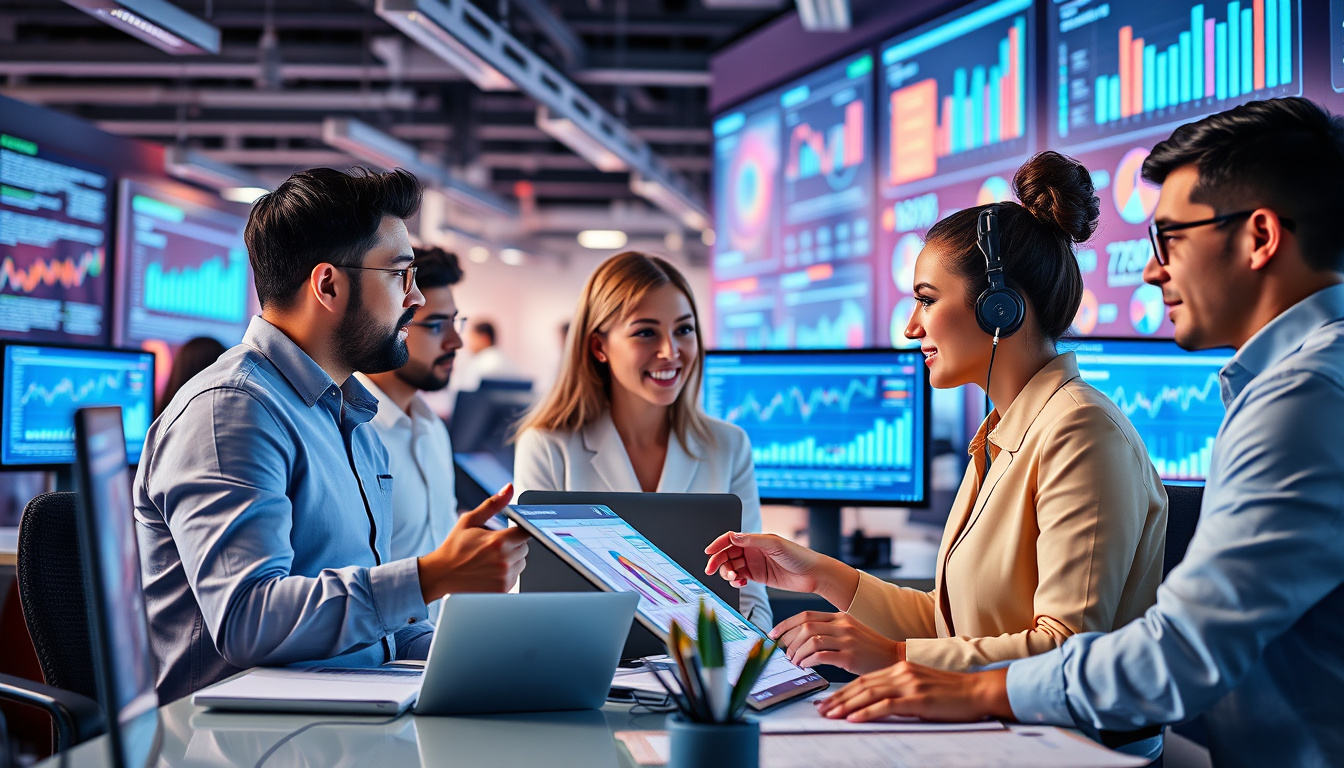
Real-World Examples of Predictive Analytics in Marketing
In todayโs competitive landscape, businesses are increasingly turning to ๐ predictive analytics in marketing explained simply to drive their strategies and enhance customer engagement. One notable real-world example can be seen in the retail sector, where companies like Amazon utilize predictive analytics to recommend products to their users based on browsing history and previous purchases. This not only enhances the shopping experience but also significantly increases sales conversions. Similarly, Netflix employs predictive modeling to analyze viewing habits and recommend shows or movies tailored to individual preferences, which keeps users engaged and subscribing to their service longer. Another example comes from the hospitality industry; hotels use predictive analytics to forecast demand patterns and optimize pricing strategies, ensuring they attract guests while maximizing revenue. These case studies illustrate how predictive analytics transforms marketing strategies and decision-making processes, enabling brands to effectively target their audience while boosting return on investment.
Challenges and Limitations of Predictive Analytics
Predictive analytics in marketing explained simply reveals how businesses leverage data to forecast customer behavior and enhance decision-making. However, like any powerful tool, it presents its own set of challenges and limitations. One primary challenge is data quality; without accurate and clean data inputs, the predictions generated can lead to misguided strategies. Another significant limitation pertains to the complexity of algorithms used in predictive modeling, which can sometimes make insights difficult to interpret for marketing teams lacking in technical expertise. Additionally, over-reliance on historical data can lead to missed opportunities in rapidly changing market conditions. Addressing these challenges is key for marketers looking to effectively harness predictive analytics in their campaigns.
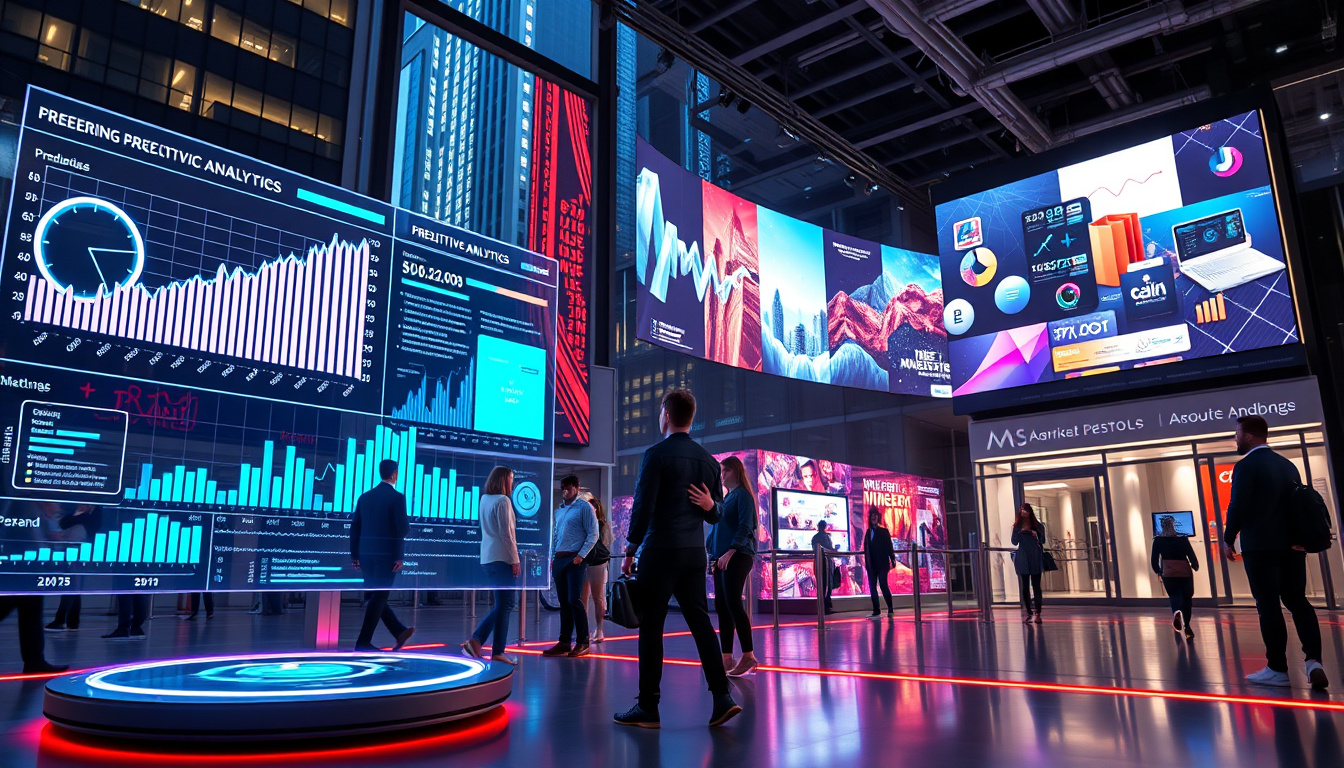
Future Trends in Predictive Analytics for Marketing
As businesses strive to keep pace with rapid technological advancements, the future trends in predictive analytics for marketing are increasingly transformative. ๐ Predictive analytics in marketing explained simply involves leveraging data from past consumer behavior to forecast future purchasing patterns, enabling brands to enhance their marketing strategies effectively. One notable trend is the rise of machine learning algorithms, which can analyze vast datasets far beyond human capability, allowing for more nuanced insights into customer preferences. Moreover, personalization is set to reach new heights; brands will use predictive analytics to deliver tailored messages based on individual consumer journeys, significantly improving customer engagement. Another key trend is the integration of predictive analytics with artificial intelligence (AI), enabling real-time data processing and decision-making, which is crucial for addressing rapidly changing market conditions. Additionally, as privacy concerns grow, businesses will likely adopt more transparent data collection practices while still harnessing the power of predictive analytics to maintain competitive advantage. By keeping abreast of these trends, marketers can better anticipate consumer needs and drive business growth.
Frequently Asked Questions
What is predictive analytics in marketing?
Predictive analytics in marketing refers to using statistical techniques and algorithms to analyze historical data and make predictions about future consumer behavior. This allows businesses to target their marketing efforts more effectively.
Why is predictive analytics important for businesses?
Predictive analytics is crucial for businesses as it helps them understand customer preferences, improve marketing efficiency, increase sales, and enhance customer satisfaction by anticipating needs and behaviors.
How does predictive analytics work?
Predictive analytics works by collecting data, applying statistical algorithms and machine learning techniques, and generating predictive models. These models analyze trends and patterns in the data to forecast future outcomes.
What are some tools and techniques used in predictive analytics?
Common tools and techniques in predictive analytics include data mining, regression analysis, machine learning algorithms, and software platforms like IBM SPSS, SAS, and Microsoft Azure.
What are the challenges of implementing predictive analytics in marketing?
Challenges include data quality issues, the need for skilled personnel, integration of predictive analytics tools with existing systems, and ensuring privacy and compliance with regulations.
Authored by - Abdulla Basha
Email id - mail@abdullabasha.com
Linkedin